What is the meaning of data housing? What is data housing examples?
Data warehousing is a systematic process of collecting, storing, managing and organizing large amounts of structured and sometimes unstructured data from various sources within an organization.
What is the meaning of data housing? What is data housing examples?
Data Warehousing – Unlocking Insights from Information
Meaning of Data Warehousing
Data warehousing is a systematic process of collecting, storing, managing and organizing large amounts of structured and sometimes unstructured data from various sources within an organization. The primary goal is to provide a centralized and integrated repository for data that can be easily accessed and analyzed. It facilitates effective decision making and reporting, as it allows businesses to gain valuable insights from vast pools of information.
At its core a data warehouse is more than just a storage facility. It involves a structured approach to data management by transforming raw data into a format conducive to analysis and reporting. This transformation process, often referred to as ETL (Extract, Transform, Load), ensures that data is cleanly standardized and integrated into the data warehouse making it suitable for analytical processing.
Major components of data warehousing
1. Extract Transform Load (ETL)
Citation raw data is gathered from a variety of source systems, including databases, spreadsheets, and external APIs.
Transformation Changes data within the data warehouse to ensure consistency, accuracy, and compatibility.
Load –The processed data is loaded into the data warehouse where it becomes available for analysis.
2. Data Warehouse Database
Central Repository – Data warehouse databases act as a central repository for structured, clean and integrated data from diverse sources.
Optimized for analytics – Unlike transactional databases, data warehouses are optimized for analytical processing, complex queries, and reporting.
3. Data Mart
Specialized subgroups – Data marts are subgroups of data warehouses focused on specific business units or departments. They provide optimized datasets for more targeted analysis.
Improved performance – Data marts can increase performance by focusing on a specific set of data relevant to a particular business function.
4. Metadata Repository
Data about data – Metadata is important in a data warehouse which provides information about the origin, meaning and structure of the stored data.
Enhances understanding – Metadata enhances the understanding of data by helping both technical and non-technical users interpret and use the information effectively.
5. Query and Reporting Tools
User-friendly interface – These tools provide a user-friendly interface to enable users to interact with the data warehouse, create reports and execute queries.
Visualization Advanced reporting tools often include data visualization features to present insights in an understandable and attractive way.
6. Data Quality and Governance
Data Cleaning – Data warehousing involves the processes of cleaning and standardizing data to maintain quality and consistency.
Governance Policies – Establishing governance policies ensures that data within the warehouse adheres to compliance standards and organizational rules.
7. Historical Data Storage
Time-based dimensional modeling – Many data warehouses use time-based dimensional modeling to store historical data. This enables analysis of trends and changes over time.
Temporary queries -Historical data storage supports temporary queries, allowing users to track changes and assess performance over specific periods.
Example of Data Warehouse
In this scenario a data warehouse can be implemented to consolidate and analyze this diverse set of data. Let's break down the key components and functionalities of a data warehouse in this retail example
1. Source System Integration
Data extraction – Sales data from point-of-sale systems, customer information from online platforms, inventory data from warehouses, and marketing data from various campaigns are extracted.
2. ETL Process
Data transformation – The extracted data undergoes transformations to ensure consistency in format, units and terminology. For example, standardizing product names and assortments across different channels.
Data Loading – The transformed data is loaded into the data warehouse creating an integrated and standardized dataset.
3. Data Warehouse Database
Centralized repository – Data warehouse serves as a centralized repository for integrated data. It contains tables of sales customer product inventory and marketing along with the relationships established between these entities.
4.Data Mart
Sales Data Mart – A data mart created to focus exclusively on sales-related data. This includes information about products sold, revenue generated and customer demographics.
Marketing Data Mart – Another data mart can be set up to satisfy marketing-related queries and provide insight into campaign performance, customer responses and returns on investment.
5. Metadata Repository
Definition of metrics – Metadata includes definitions of metrics such as total sales, customer acquisition cost, and inventory turnover. This helps to understand the meaning and context of each data point.
6. Query and Reporting Tools
User-friendly interface – Business analysts and executives can use query and reporting tools to generate reports on sales performance, track inventory levels, and assess the impact of marketing campaigns.
7. Data Quality and Governance
Data Cleaning – The data warehouse implements processes to clean and validate incoming data while ensuring accuracy and reliability.
-Governance Policies – Policies are established to control data use, define who has access to specific information and ensure compliance with industry regulations.
8. Historical Data Storage
-Time-based analysis – Historical data is stored to enable time-based analysis. This allows the company to assess sales trends across different seasons, evaluate the success of marketing campaigns over time, and plan for future inventory needs.
conclusion - a data warehouse becomes a strategic asset that provides a comprehensive view of a company's operations. It enables informed decision making, supports strategic planning and enhances overall business intelligence by transforming raw data into actionable insights.
What's Your Reaction?
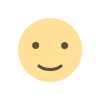
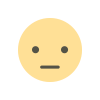
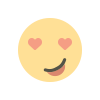
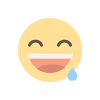
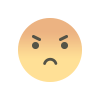
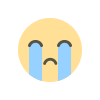
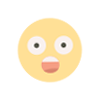