what is data science
The main objective of data science is to extract meaningful insights and knowledge from data to inform decision making, solve complex problems and promote innovation in various domains.
what is data science
Data science
The main objective of data science is to extract meaningful insights and knowledge from data to inform decision making, solve complex problems and promote innovation in various domains. Data scientists use a combination of statistical analysis, machine learning techniques, programming skills and domain expertise to uncover hidden patterns, trends and correlations within large and diverse datasets.
By harnessing the power of data, organisations can optimise processes, enhance products and services and gain a competitive edge in the marketplace. Data science enables businesses to better understand their customers' behaviour preferences and needs leading to more targeted marketing strategies and personalised experiences.
In areas such as health care data science facilitates disease diagnosis and prognosis drug discovery and patient care optimization through predictive analytics and data-driven insights. Additionally data science plays an important role in fields such as finance, transportation energy and environmental science where it helps in risk management, resource optimization and sustainability efforts. Overall the main objective of data science is to strategically leverage data as an asset empowering decision makers to make informed choices foster innovation and create value in a data-driven world.
Do we need Data Science?
We need data science for several reasons
1. Data-Driven Decision Making Data science helps organisations make informed decisions based on empirical evidence rather than intuition or guesswork.This leads to more accurate predictions and better results.
2. Insight Discovery Data science uncovers valuable insights and patterns hidden within large and complex datasets that would otherwise have been overlooked. These insights can drive innovation, optimise processes and identify new opportunities.
3. Problem Solving Data science provides powerful tools and techniques to solve complex problems in various domains ranging from healthcare and finance to marketing and transportation.By analysing data, data scientists can solve challenges more effectively.
4. Optimization Data science optimises processes systems and resources by identifying inefficiencies streamlining operations and maximising performance. This leads to cost savings improved productivity and increased competitiveness.
5. Personalization Data science enables organisations to tailor products, services and experiences to individual preferences and needs. This personalised approach increases customer satisfaction and loyalty.
6. Innovation Data science drives innovation by uncovering new insights, identifying emerging trends and fostering experimentation and creativity. It drives advancements in technology, science and business.
data science is essential to navigate the increasingly data-driven world we live in, empowering organisations to make better decisions, solve complex problems and drive progress and innovation.
types of data science
Indeed data science includes various types and subfields each with its own methodology techniques and applications.
1. Predictive Analytics - Predictive analytics involves using historical data to make predictions about future events or trends. Machine learning algorithms such as regression classification and time series forecasting are commonly used in predictive analytics.
2. Machine learning - Machine learning is a subset of data science that focuses on developing algorithms and models that can learn from data and make predictions or decisions without being explicitly programmed. This includes supervised learning, unsupervised learning and reinforcement learning.
3. Deep Learning - Deep learning is a special form of machine learning that involves neural networks with multiple layers (deep neural networks).
4. Natural Language Processing (NLP) - NLP is a branch of data science that focuses on understanding and generating human language. It includes techniques such as text mining sentiment analysis, machine translation and named entity recognition.
5. Computer Vision - Computer vision is the field of data science that teaches computers to interpret and understand visual information from the real world. It includes tasks such as object detection, image classification and image segmentation.
6. Big Data Analytics - Big Data Analytics deals with the analysis of large and complex datasets that exceed the processing capabilities of traditional data processing applications. It includes distributed computing frameworks such as Hadoop and Spark and technologies for data storage retrieval and analysis.
7. Time Series Analysis - Analyzing data collected over time to uncover patterns and trends.
8. Network Analysis - Examining the connections and relationships between entities in a network, such as social networks or transportation networks.
These are just a few examples of the different types in the field of data science each with its own set of techniques and applications tailored to different data-driven challenges and opportunities.
What's Your Reaction?
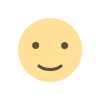
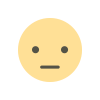
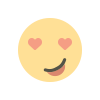
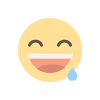
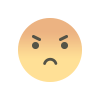
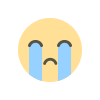
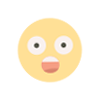